Deep Fools
New Electronics has an article that includes my Deep Learning and Security Workshop talk: Deep fools, 21 January 2019.
A better version of the image Mainuddin Jonas produced that they use (which they screenshot from the talk video) is below:

ICLR 2019: Cost-Sensitive Robustness against Adversarial Examples
Xiao Zhang and my paper on Cost-Sensitive Robustness against Adversarial Examples has been accepted to ICLR 2019. Several recent works have developed methods for training classifiers that are certifiably robust against norm-bounded adversarial perturbations. However, these methods assume that all the adversarial transformations provide equal value for adversaries, which is seldom the case in real-world applications. We advocate for cost-sensitive robustness as the criteria for measuring the classifier’s performance for specific tasks.Can Machine Learning Ever Be Trustworthy?
I gave the Booz Allen Hamilton Distinguished Colloquium at the University of Maryland on Can Machine Learning Ever Be Trustworthy?. [Video](https://vid.umd.edu/detsmediasite/Play/e8009558850944bfb2cac477f8d741711d?catalog=74740199-303c-49a2-9025-2dee0a195650) · [SpeakerDeck](https://speakerdeck.com/evansuva/can-machine-learning-ever-be-trustworthy) Abstract Machine learning has produced extraordinary results over the past few years, and machine learning systems are rapidly being deployed for critical tasks, even in adversarial environments. This talk will survey some of the reasons building trustworthy machine learning systems is inherently impossible, and dive into some recent research on adversarial examples.Center for Trustworthy Machine Learning
The National Science Foundation announced the Center for Trustworthy Machine Learning today, a new five-year SaTC Frontier Center “to develop a rigorous understanding of the security risks of the use of machine learning and to devise the tools, metrics and methods to manage and mitigate security vulnerabilities.” The Center is lead by Patrick McDaniel at Penn State University, and in addition to our group, includes Dan Boneh and Percy Liang (Stanford University), Kamalika Chaudhuri (University of California San Diego), Somesh Jha (University of Wisconsin) and Dawn Song (University of California Berkeley).Artificial intelligence: the new ghost in the machine
Engineering and Technology Magazine (a publication of the British [Institution of Engineering and Technology]() has an article that highlights adversarial machine learning research: Artificial intelligence: the new ghost in the machine, 10 October 2018, by Chris Edwards. Although researchers such as David Evans of the University of Virginia see a full explanation being a little way off in the future, the massive number of parameters encoded by DNNs and the avoidance of overtraining due to SGD may have an answer to why the networks can hallucinate images and, as a result, see things that are not there and ignore those that are.USENIX Security 2018
Three SRG posters were presented at USENIX Security Symposium 2018 in Baltimore, Maryland: Nathaniel Grevatt (GDPR-Compliant Data Processing: Improving Pseudonymization with Multi-Party Computation) Matthew Wallace and Parvesh Samayamanthula (Deceiving Privacy Policy Classifiers with Adversarial Examples) Guy Verrier (How is GDPR Affecting Privacy Policies?, joint with Haonan Chen and Yuan Tian) There were also a surprising number of appearances by an unidentified unicorn: Your poster may have made the cut for the #usesec18 Poster Reception, but has it received the approval of a tiny, adorable unicorn?Mutually Assured Destruction and the Impending AI Apocalypse
I gave a keynote talk at USENIX Workshop of Offensive Technologies, Baltimore, Maryland, 13 August 2018. The title and abstract are what I provided for the WOOT program, but unfortunately (or maybe fortunately for humanity!) I wasn’t able to actually figure out a talk to match the title and abstract I provided. The history of security includes a long series of arms races, where a new technology emerges and is subsequently developed and exploited by both defenders and attackers.Dependable and Secure Machine Learning
I co-organized, with Homa Alemzadeh and Karthik Pattabiraman, a workshop on trustworthy machine learning attached to DSN 2018, in Luxembourg: DSML: Dependable and Secure Machine Learning.
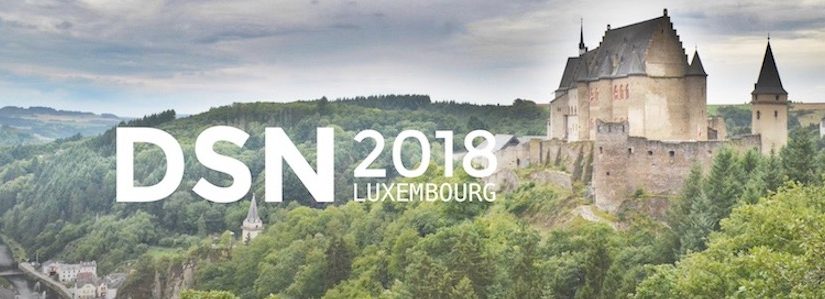